Part 1 of this two-part article defined adoption and talked about what makes it hard in any organization. Part 2 describes the ways you can manage adoption challenges.
Exec Summary: Over the past year, countless blogs, articles, books, videos, courses – even job descriptions – focused on prompts and prompt engineering. While prompting is essential to effective GenAI use, it’s only one thing to consider. Generative AI outputs are another, and they need more attention.
Recall the IPO Model – inputs, processes, and outputs. For a simple generative AI use, prompts are the inputs, algorithms are the process, and a GPT’s response is the output. For more complex uses, inputs and processes combine as a user and the GPT interact through a set of prompts. Outputs can also take on a new importance, depending where they lead.
If the outputs of my GPT use become inputs to a business task or process you own, we face added requirements for communication, collaboration, and probably change management. And that calls for an approach to addressing the hard adoption questions.
How Do We Answer The Hard Adoption Question?
Meeting the challenge of generative AI adoption will require a comprehensive and methodical approach. Here are three principles we’re applying at DWPA we recommend you consider.
- Use an adoption framework
- Clarify goals and objectives
- Think like an entrepreneur
Use An Adoption Framework
The grand-daddy of innovation adoption frameworks might be Everett Rogers’ Diffusion Innovation Theory. In his 1962 classic (updated through a 5th edition in 2003), Rogers explains how an innovation diffuses through, or is adopted by, a social system. There’s a lot to Rogers’ research and it would be worth your time to read select portions of this book. But we can highlight the pieces you can use immediately.
Most well-known might be adoption types Rogers identified and arrayed temporally in an S-curve. Rogers called it the innovation adoption customer because he studied many types of innovation. Today it’s popularly known as the technology adoption curve, as shown here:
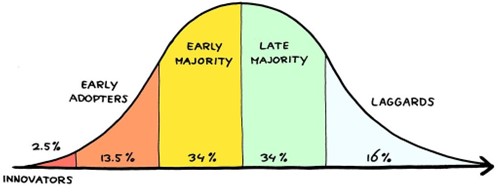
This shows that adopters organize in five types within any social system – your company, the Federal government, the govcon market, etc. – and that they adopt at different rates. This happens because of the time they take moving through six stages Rogers identified:
- Knowledge is gained when someone learns of the existence of an innovation, and gains some understanding of how it works. This leads to Persuasion.
- Persuasion occurs when someone forms a favorable or unfavorable impression of an innovation, generally before using. This leads to a Decision.
- Decision occurs when someone engages in activities which lead to adoption or rejection. When adoption occurs, this leads to Implementation.
- Implementation occurs when someone puts an innovation to work. This leads to Confirmation.
- Confirmation occurs when someone is reinforced for additional use, or reverses their decision and rejects the innovation.
Finally, adopters do all this because of the different ways they evaluate the following Innovation Adoption Factors:
- Relative advantage is the degree to which an innovation is perceived as better than the idea it supersedes.
- Compatibility is the degree to which an innovation is perceived as being consistent with the existing values, past experiences, and needs of potential adopters.
- Complexity is the degree to which an innovation is perceived as difficult to understand and use.
- Trialability is the degree to which an innovation may be experimented with on a limited basis.
- Observability is the degree to which the results of an innovation are visible.
You might be familiar with another simpler framework called the Technology Adoption Model which looks at just two factors – perceived usefulness, and perceived ease of use. You might have a preferred framework, model, or theory. The important thing is to use one (or more) so everyone works with the same concepts and terms. Without that, people who need to be on the same page won’t be.
Clarify Goals And Objectives
The second principle is to clarify both uses or use cases, and broader adoption goals and objectives. It helps to clarify uses with a statement like the following, which captures use case elements:
As a [role], I want to [perform some action] on [some artifact] to produce [some output]
in order to [accomplish something] or for [some reason].
This will not only help everyone think through any single use case, but it’ll promote uniformity and consistency across uses by all individuals, teams, and other organizational units. There are other ways to do this but the important thing, again, is that you get everyone on the same page by framing uses with concepts whose meanings are shared.
Clarifying adoption goals and objectives is trickier because adoption occurs by individuals, teams, business verticals, business functions, and the entire enterprise. Each properly has its own business-related goals and objectives which can exist in nested, prioritized, instrumental, or a number of other relationships.
Because adoption is about making full use of generative AI, and because making full use should do something better than what you’re currently doing, it’s important to use frameworks for figuring out what better means at any level. You might already use frameworks for individual performance, collaboration, productivity, innovation, or other things related to one or more levels. DWPA uses the Business Model Canvas.
Think Like An Entrepreneur
“Think like an entrepreneur” is a way to summarize DWPA’s entire GenAI Discovery Project, which we’ve written about extensively.
We’ve described our process for state assumptions about generative AI, our clients, and the govcon market and how we turned them into hypotheses to test. Test results are evidence we’re using to fashion capture and proposal generative AI-assisted services to validate with customers before going to market with them.
Generative AI is innovative and your use of it is also innovative. It helps to think like an entrepreneur because by adopting an innovation you are literally doing something different to create new value for yourself, internal recipients, and perhaps your customers.
At the outset there will be nothing but assumptions because you can’t have evidence for generative AI use you don’t have. State all the assumptions you can think of, turn important ones into hypotheses, and test them. Tests can be quick and easy – generative AI trials, simulations, if-then scenarios, voice of the customer, and more.
You need hours and days to try something to see what you get, and that’s your evidence. You’ll get strong evidence. You’ll get weak evidence. Collect it. Appraise it against goals and objectives, and apply it to see what happens in what actually amounts to another round of hypothesis testing and evidence gathering.
Conclusion
Generative AI is a powerful technology which is changing the human-machine relationship. And that has the potential to change the human-human relationship. Whether that change is beneficial or not depends entirely on us.
Use generative in the way you use all other software and you’ll get some ROI but not what you could get. Shift your thinking from use to adoption and you’ll not only execute tasks faster, you’ll improve communication, collaboration, and problem solving.