December 2023 – Vol. 12; Issue 12
DWPA Staff
on
December 28, 2023
Generative AI: The Easiest New Year’s Resolution You Can Keep
- Generative AI made big news in 2023 and it promises to make more in 2024, so it is time for everyone to learn what the hype is about
- In time, generative AI will be in all business applications, so it is important to try it sooner rather than later
- Many companies have already adopted the countless benefits of generative AI, don’t fall behind on learning how you can benefit from it
- Trying generative AI for yourself is by far the best way to see what it can do for you.
- Learn the differences between public web-based tools and licensed private tools
- Exploring generative AI could be the most fun, and possibly the most profitable, 2024 New Year’s Resolution you make
The Hottest Resolution for 2024
Every GovCon business runs on basic software for productivity, accounting, security, and more. Some run specialized software. Some sell software-related products and services. Computer software is as central to business, today, as ledgers and typewriters once were. We also know software will advance. We know we’ll have to keep up, and we try not to be hasty. Deliberation is good and caution can be warranted. Companies and entire industries have adoption patterns. And that leads us to generative AI (GenAI). Owners and Execs are cautious of generative AI, if not skeptical. It’s very new, and it works differently than most, if not all, other business software. Its programmers don’t know exactly how it does what it does. It’s known to fabricate information. It might make your proprietary information public. Agencies’ views are mixed. It’s hyped to change the world, so caution seems warranted.
Follow Through on Your Resolution
Generative AI is unambiguously beneficial. Need a draft to get you started? Technical content simplified? Resumes found and scored? Done, done, and done. Generative AI can help in dozens of ways on client projects, in the back office, and in business development, capture, and proposals. It holds the promise not only of increased effectiveness and efficiency, but also of improved competitiveness. So, if you don’t use GenAI but your competitors do, that might cost you. For innovative technology, generative AI is inexpensive, easy to use, easy to scale, and improves with use. Its “black box” is fascinating or worrisome, depending on your point of view. So how do you make this risk-benefit decision?
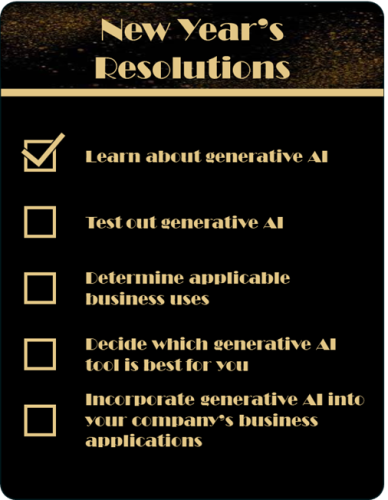
Planning Out Your Resolution
Choose a safe, easy starting point for your first try. Especially when using a public, Web-based tool, ask about subjects of general interest, technical questions you usually Google, news stories, or published reports. See what you get, pick part of the answer, and ask more about it. Challenge part of an answer. Ask it to answer at a fifth-grade level. Or summarize. Or elaborate. See what you get and ask conversational follow-on questions, just like when you talk to a subject matter expert. Your goal is to gain some knowledge of generative AI’s capability by using it, so you can see the potential for more organized and targeted use. Unlike some New Year’s Resolutions which are difficult to keep, this one might inspire you to do more.
Exercise Safe Practices when Starting Your Resolution
You have choices about where to start. Ask a vendor for a trial license or try a publicly available, Web-based tool like ChatGPT, Bard, Claude, or Bing. If Microsoft’s Co-pilot is available in your productive applications, you can use it. Choose a tool to which you have easy access and have a go. You can compare and contrast, later. For any tool you choose, read the vendor’s data, use, or privacy policy so you know what happens to your content. You especially want to know if your content is included in future tool training. By reading the company’s policy you’ll also know if you can opt out of certain uses of your content, such as for training. If using a public tool, don’t enter content which is privileged in any way. There’s more to know about safe use but if you avoid privileged information for your first use, you limit your risk. If you use a licensed private tool, you can be more confident using proprietary information. Still, read and ask about the vendor’s data and privacy policies.
Six Pieces of Advice when Starting out with Generative AI
- If you’ve been avoiding generative AI until now, do something with it. The more you try it, the more you’ll see business uses.
- Start small. Start safe.
- Don’t think of generative AI like a search engine. Think of it like a smart colleague or consultant and interact with it the way you’d interact with them.
- Be curious. If you wonder if generative AI can do something, ask it. Ask it something general, specific, or even fanciful.
- If your prompt is lengthy or complicated, chunk it down to smaller pieces.
- Know your tool’s data, use, or privacy policy, and exercise caution adding privileged information to tools which train on user inputs.
Lou Kerestesy
703-835-3267
lou.kerestesy@dwpassociates.com
TJ Sharkey
202-591-5958
thomas.sharkey@dwpassociates.com
Doug Black
703-402-4511
doug.black@dwpassociates.com