Work teams usually contain members of different types. Some are risk taking, others risk averse. Some think big picture, others work in the weeds. You have introverts and extroverts, different DiSC profiles, and more.
When people think, feel, and communicate accordingly, getting everyone on the same page can be a challenge. This is especially true of change, and that presents a special challenge for adopting generative AI (GenAI).
How do you help different types try and use generative AI so individuals, teams, and your organization benefit in ways you intend?
If you don’t have time to sort all this out, we’ll get you started. This article highlights two adoption frameworks and shows you how to put them to work, together.
Follow this page for weekly insights, and contact Lou.Kerestesy@DWPAssociates.com for more information.
A GenAI Challenge And Opportunity
Individuals and teams face known challenges adopting innovations. One 2015 National Library of Medicine study examined 20 adoption theories and frameworks, some of which have become well-known over time. One is the innovation adoption curve, popularly known as the technology adoption curve. Another is the technology acceptance model. More on both below.
What’s less well-known is how to support adoption by type. We have less research on this, and much of what’s available is written by typology advocates. With different types on our teams, this presents a generative AI adoption challenge.
When we talk about GenAI at work, we might expect colleagues or team members to hear one conversation. The same conversation. We might not consider that different types hear the conversation in different ways. That they think and feel their way through adoption in ways that make sense to them – and that there isn’t one way for all types.
So, if frameworks tell us the types of questions adopters ask, how do we help types of adopters answer them, together? How do we help individuals and teams have a collective conversation about generative AI without talking past one another?
Let’s look at the two frameworks we mentioned to see if they’ll help.
Framework 1: The Technology Acceptance Model
Developed in the 1980s, the Technology Acceptance Model (TAM) explains how users come to accept and use technology. It identifies two primary acceptance considerations – Perceived Usefulness and Perceived Ease of Use.
Perceived Usefulness refers to how much one believes a technology will enhance performance. Perceived Ease of Use refers to how much effort one believes will be required. TAM proposes that these two perceptions affect one’s intent to use a technology and that, in turn, affects one’s actual use.
One general finding is that Perceived Ease of Use affects Perceived Usefulness. The easier a technology is perceived to be, the more it’s seen as enabling better performance. The four-cell matrix is a common representation among these basic relationships.
Framework 2: The Diffusion of Innovation Theory
Developed in the 1960s, the Diffusion of Innovation Theory explains how innovation spreads through systems to be adopted or rejected. Like TAM, the Diffusion of Innovation Theory has been widely researched and popularly used.
The theory’s original author, Everett Rogers, investigated how innovations diffuse through social systems ranging from businesses to agrarian tribes. There are few adopters of any innovation at first, then more, then many, and over time adoption levels off. Adoption follows an S-shaped curve and Rogers identified five adopter categories along it:
- Innovator
- Early Adopter
- Early Majority
- Late Majority
- Laggards
Rogers called this the innovation adoption curve, but today it’s well-known as the technology adoption curve. Rogers also hypothesized that adopters proceeded through five adoption stages:
- Knowledge – Knowledge is gained when an individual learns of an innovation’s existence, and gains some understanding of how it functions
- Persuasion – Persuasion takes place when an individual forms a favorable or unfavorable attitude toward an innovation
- Decision – Decision occurs when an individual engages in activities that lead to a choice to adopt or reject an innovation
- Implementation – Implementation takes place when an individual puts an innovation to use
- Confirmation – Confirmation occurs when an individual seeks reinforcement of their decision to use an innovation, or reverses that decision if exposed to conflicting information
Rogers, Everett M.. Diffusion of Innovations, 5th Edition (p. 23). Free Press. Kindle Edition.
Combining Frameworks
If we create a matrix using both framework’s key elements, we provide each adopter a way to think about and document their thoughts, feelings, hopes and dreams with regard to GenAI adoption:
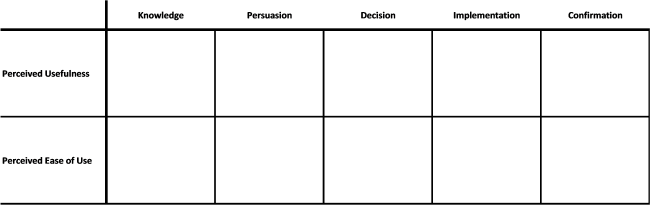
In it, any user can document the information they look for to judge usefulness or ease of use (knowledge), what would make them form a favorable or unfavorable view of GenAI’s perceived usefulness or ease of use (persuasion), etc. Because each user would fill in the table, their comments would represent their voice as whatever type they happen to be.
Individuals, teams, or entire organizations could identify types if that were considered useful, but doing so isn’t necessary. If each person records their rationale in each cell, it gives colleagues, teams, business units, and organizations a basis for understanding perceived usefulness and perceived ease of use from multiple, diverse, perspectives.
That should be enough for differences related to types to benefit conversation, without making a study of types.
Conclusion
We want conversations to “get at” the different ways colleagues and team members think about generative AI, and excavating views by type is especially valuable for two reasons.
First, generative AI is available to your organization as general-purpose apps, domain-specific apps, and GPTs you create. Not only do you need to evaluate GenAI as a capability, you need to evaluate the form or forms in which individuals and teams will adopt and use it. It could be very instructive to see if different types prefer different forms.
Second, as organizational change goes, generative AI might be especially weighty because of its expected impacts to jobs and performance. Some will wonder, “Will my job change? Will I be able to change with it? Will my job go away?” Others might think, “We could use GenAI to improve so much – but we’re dragging our feet!”
To whatever degree views of GenAI’s change impacts depend on types, it would help you make adoption and investment decisions to know not just that certain individuals or teams view GenAI the way they do, but why they do. Especially if other individuals or teams view GenAI in the opposite way. Conversations about GenAI adoption with voices by type will help get you there.
Follow DWPA’s company page for weekly discovery insights. To learn more or launch your own discovery project, contact Lou.Kerestesy@DWPAssociates.com.